How emotional AI bots can be used in customer services
Im Beitrag geht es um Technologien zur Emotionserkennung im Kundenservice.
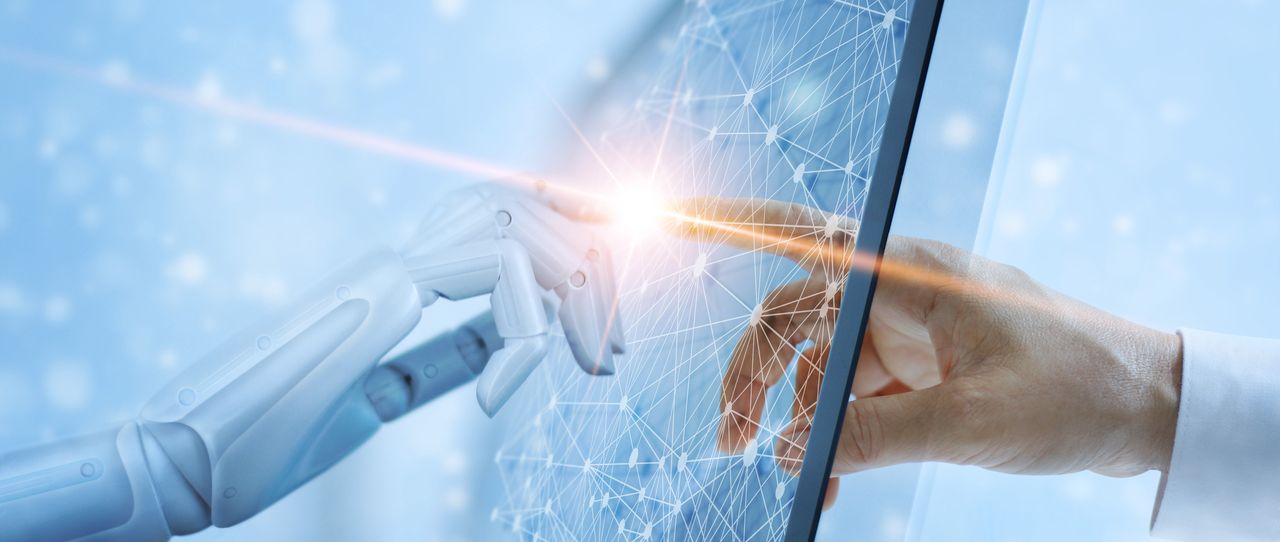
Customer service interactions empowered by Artificial Intelligence have shown a great impact on efficiency, productivity and overall customer relationship management. Introducing automatic steps to handle user’s requests has greatly reduced waiting time in queue through different channels.
Traditionally, customer engagement uses channels like email, telephone and local agents. In 2016, chatbots emerged as a new trend, making it a topic of interest for companies eager to stay connected with their customers. Conversational agents powered by AI could be plugged in any of these channels to receive the request, understand it using a Natural Language Processing engine and process it with the business-related knowledge. Using these powerful tools has given promising results in theory. However, consumers can often be left disappointed by their encounters with the technology, as user requests can be misinterpreted or simply met with a lack of empathy.
Therefore, computer scientists are increasingly interested in adding an affective component to the human-agent interaction systems. A new research area is then emerging : affective computing which is often called emotional AI.
This field convey numerous applications : emotion recognition in text / voice/ image, sentiment analysis, emotion classification, affective dialog management, emotion displaying in avatars, emotion modeling and regulating. These applications share a common goal : recognizing, understanding and reasoning on human emotional mechanisms.
Automatic Emotion detection
Numerous industries are using emotion detection techniques to have an overview on their customers' feelings towards provided services or products. Sentiment Analysis is a text-based approach applied to customers written feedback on social media, chatbots or websites in order to extract a polarity (negative or positive). Technically, customers’ words are first represented as vectors (embeddings) before feeding into a classification model which gives them a label (positive or negative) and a confidence score. Recently, transformer-based language models have shown a great performance as they are pre-entrained with a huge amount of unlabeled data making the need of labeled data lower. The classification task could be more accurate if we use emotion labels rather than sentiment labels. This task is more challenging as a customer input could convey multiple emotions.
Some AI solutions are using the voice as a main source of real-time feedback. Indeed, it has been proved that the vocal signal reveals richer emotional information than the verbal content. The user’s signal is transformed to a spectrogram and then fed into a deep neural network. The output is an emotional label and a confidence score.
The work done by the Zaion team in this area has given promising results : 91% of accuracy on sentiment text classification and 87% in anger detection in voice. We aim at capturing more customer services-related emotions : frustration, dissatisfaction, satisfaction, etc.
Affective machines
Capturing emotional patterns in users’ utterances is a first step towards an affective conversational bot. It has been validated that machines which express affective states enhance the user’s satisfaction and commitment (Prendinger and Ishizuka, 2005). This role of affects is crucial for conversational agents that have to combine a rational answers with social behaviours. For instance, when the user expresses disappointment about a task done by the agent, a credible agent should be inclined to apologize rather than pursuing the task-oriented interaction. However, combining task-oriented answers with socio-affective behaviour in a dialogue is still challenging.
Conversational AI is now employing emotion to enhance the credibility of an interaction, enabling consumers to build a social relationship with bots. It may sound surprising, but some bots even express a personality and this is shown to have a more positive impact on the customer experience. Amazon Alexa now has an emotional engine which has improved user satisfaction by 30%. For example, consumers can now ask Alexa how their favorite team has performed in a recent game and expect an enthusiastic response from Alexa if their team has won.
Emotion intensity should be context-dependent when introducing a personality model, with some bots more extroverted than others. The bot should capture emotional content from the user and respond accordingly. Emotional intensity is also environment-dependent. Typically, bots who interact with customers in sales should show more excitement and positivity, as sales agents do.
Expressing context-incoherent emotions could be dangerous of the user experience: showing an excitement while the user is frustrated could be harmful. Emotional AI must be trustworthy.
The way forward is clear. Companies should explore and adopt emotional AI in order to take advantage of all its benefits. However, in order to avoid incoherent situations, affective bots should be built with a context and environment awareness.